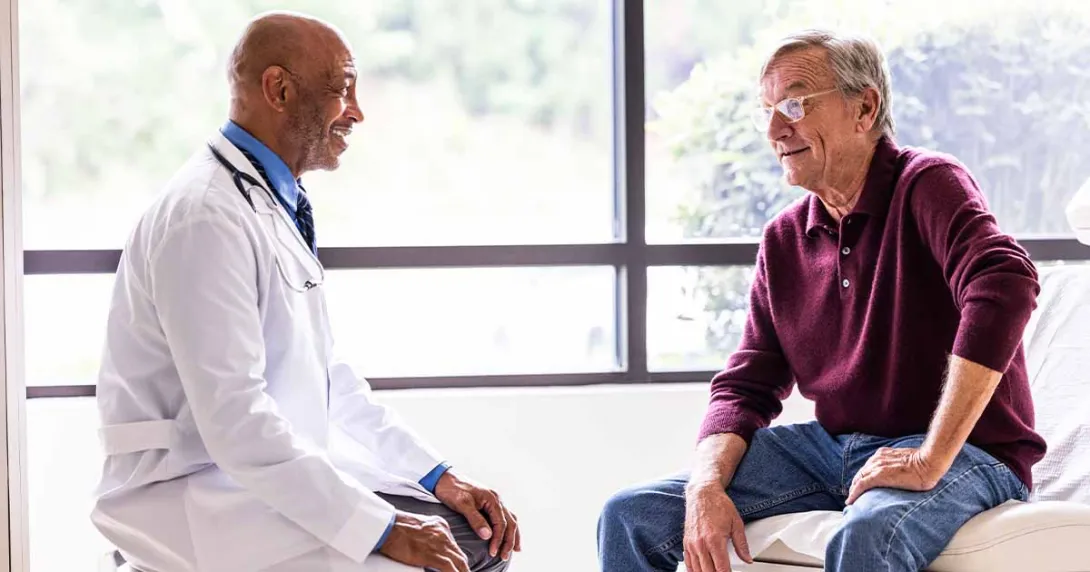
Generative AI (Gen AI) is already delivering improvements in patient experiences and health outcomes, as well as easing administrative burdens for doctors, nurses and clinicians. With the demand for healthcare outpacing the workforce, healthcare organizations need help from AI tools and automation.
At the same time, the deployment of AI technologies is rarely plug and play. The list below aggregates the most common roadblocks and concerns many organizations have encountered, along with a few ideas on how to help work through — or around — them.
1. Lack of trust
Does your team understand Gen AI’s capabilities? One concern is simply that, even if an AI implementation goes well, employees and patients may not trust it enough to use it. This concern is warranted; while AI has been around a long time, Gen AI and agentic AI are still relatively new technologies, and people might not have the information they need to be confident and comfortable with them.
Trust is all about context. Patients and providers may not want AI systems to make major patient-care decisions, but may approve of using AI to summarize clinical notes, offer decision support or generate a first draft of a patient visit summary. Right-size use cases to align with governance policies and company goals. Throughout the journey, AI users still need to be accountable for any use of AI-generated content or recommendations.
One promising initiative to improve trust in AI for healthcare organizations is the Coalition for Health AI (CHAI™). The coalition is a key leader in the drive to establish standards for the responsible use of AI in healthcare and a valuable source of guidance for healthcare systems of all sizes, wherever they are in their AI journey.
2. Concerns about accuracy
If you’re using Gen AI to provide information, you need to ensure the data going into the tool is accurate and that reliable AI algorithms are in place to guide the best outcomes. Whether you’re using AI to provide information, generate content, make recommendations or take action of some kind, human oversight and continuous monitoring should be in place to ensure accurate responses and continued trust among stakeholders.
The specific use case in question will determine the level of risk involved, which, in turn, will determine the level of monitoring and oversight required. Most Gen AI tools have become better at referencing sources, making it easier to verify any content or responses they generate. Humans must remain in the loop to make these evaluations.
3. Staff training
Adopting AI tools may elicit a spectrum of reactions rooted in perceived risks and unfamiliarity. This fear is real, and providing guidance and information to your team about AI is essential. Just like reading the instruction manual for a new car before operating it, teams expected to use AI should be trained on proper usage. Policies and guidelines to govern AI’s use should be created after gathering input from stakeholders across the organization.
AI use cases involving patients should be closely scrutinized, monitored and properly evaluated for risk to reduce the chance of detrimental outcomes for patients, employees or other stakeholders. Patient care must always come first. With that in mind, there may be some use cases to avoid because they would make key stakeholders uncomfortable, and others that have the potential to deliver enough benefit that they can be implemented with close monitoring and supervision.
Healthcare personnel must have realistic expectations about what AI can and cannot do, as well as the facts and knowledge to articulate those expectations clearly to all stakeholders.
4. Protection of intellectual property
Just as travelers must respect traffic laws, AI users should have policies and guardrails in place to guide AI use of copyrighted material. Concerns about copyrighted material usually fall into two categories: (1) the possibility of inadvertently infringing upon existing copyrights and (2) the ability to copyright new material generated by AI. In both cases, users should seek and follow guidance from their organization’s legal team when producing any content, including research, that they would typically consider intellectual property. In general, content created by AI tools should be seen as providing helpful first drafts to be reviewed and modified by the user. This also helps avoid replicating anyone’s existing work.
Each issue deserves close attention and action to mitigate risks and concerns. A comprehensive AI governance program with training and policies can help guide responsible and successful AI implementations.
To learn more, visit Human-centric AI research: A study in employee trust and workplace experience.